Learning Tight Bounds on BER to Improve Deep Neural Network Preformance
Large networks such as social networks and biological systems are common challenges in many fields such as Machine Learning, Bioinformatic, Biology, Statistics, and Computer science. The connectivity of these networks/systems is usually unknown and has to be learned from the observational data. Many important problems in these natural and social sciences involve high-dimensional directed acyclic graphs (DAGs) estimation. Under this umbrella, we develop a framework which essentially incorporates fast graph-based dependency criterion to estimate the skeleton of the large networks. We specifically are seeking approaches that previous imposed assumptions, like linear structure equation model, are relaxed. We study the empirical and structural consistency of our proposed algorithms. In this project novel DAGs methods are extensively explored on real biological data-sets.
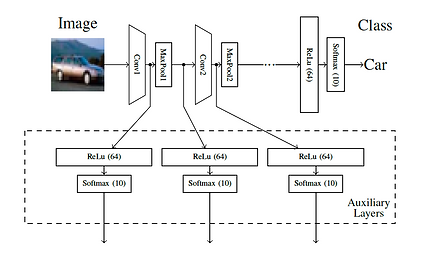
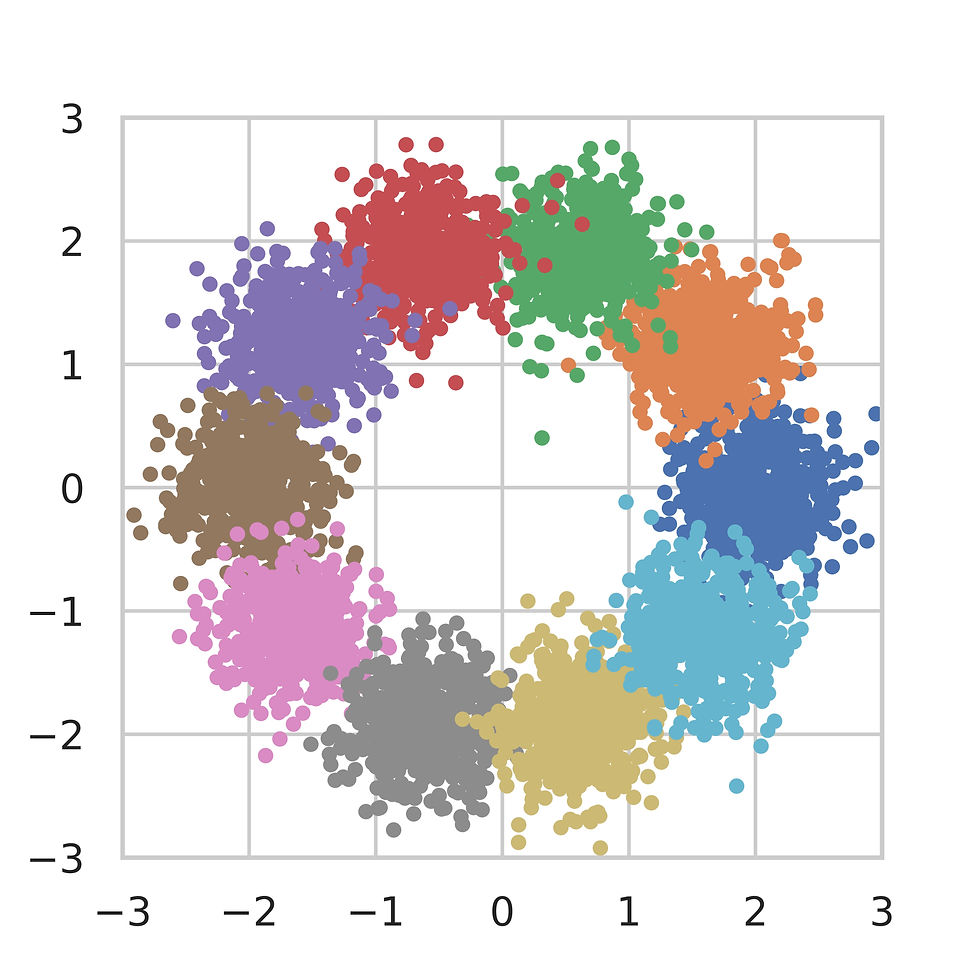
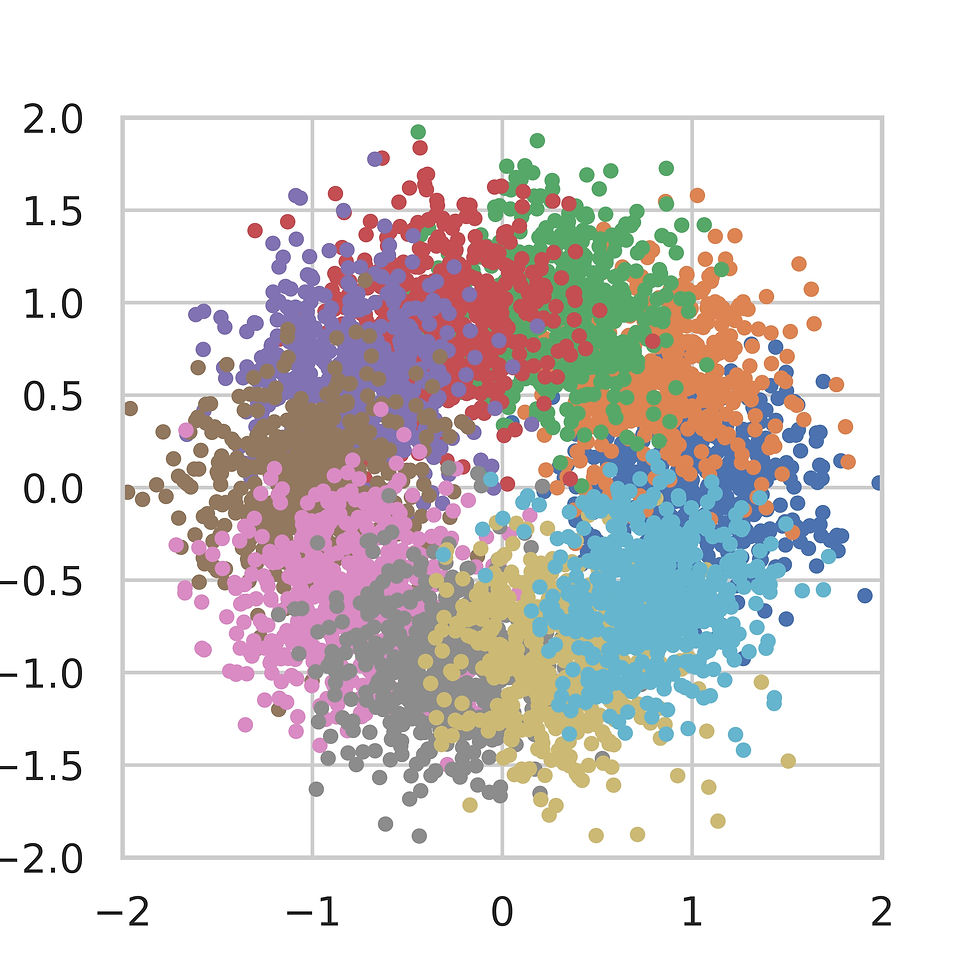
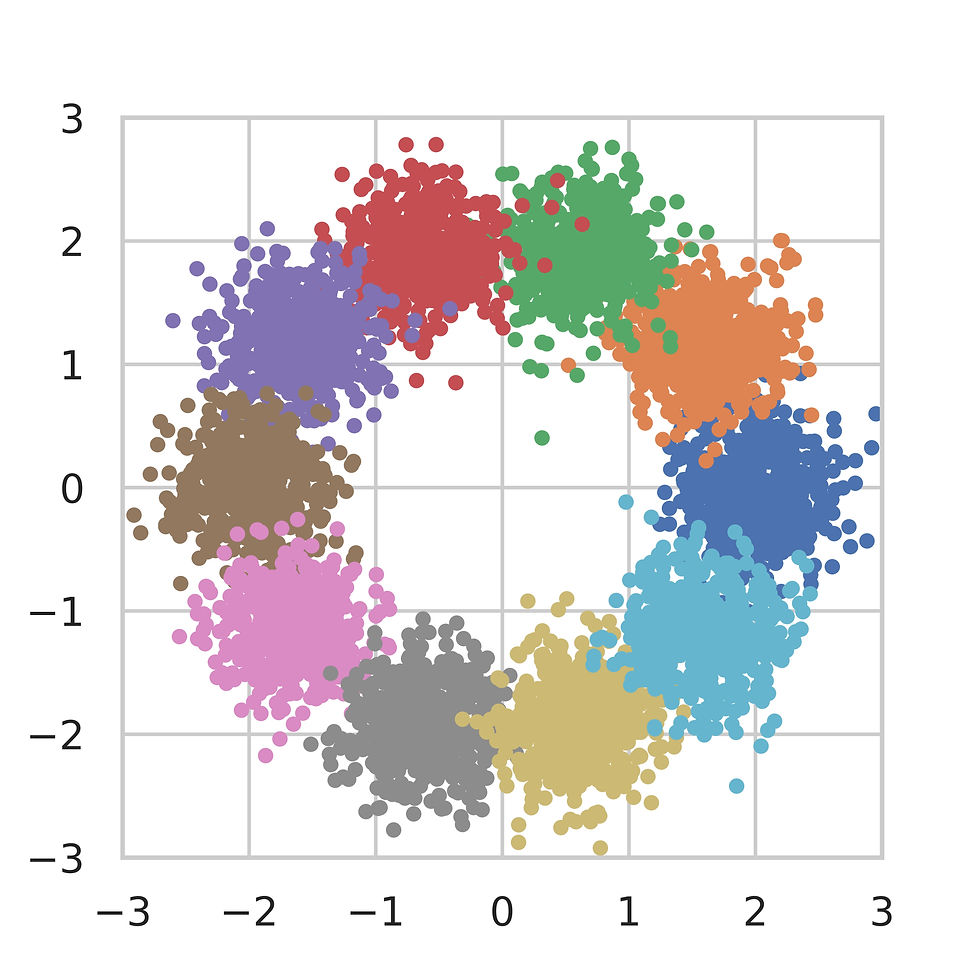