top of page
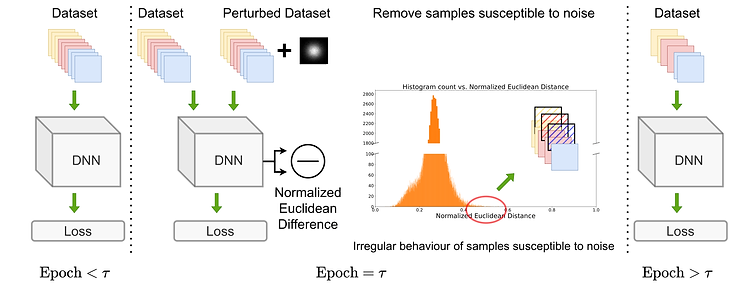
Deep Network Performance, Efficiency, Robustness
Adversarial robustness of deep neural networks has been studied extensively and can bring security against adversarial attacks/examples. However, adversarially robust training approaches require a training mechanism on the entire deep network which can come at the cost of efficiency and computational complexity such as runtime.
Increasing performance has been the de facto emphasis when developing DNN-based solutions. However, to deal with the rigors of the real world, DNNs should not only be 1) highly accurate, but 2) efficient to develop, and 3) robust to adversaries as well. We investigate to concurrently target Performance, Efficiency, and Robustness, and ask just how far we can push the envelope on simultaneously achieving these goals.
bottom of page